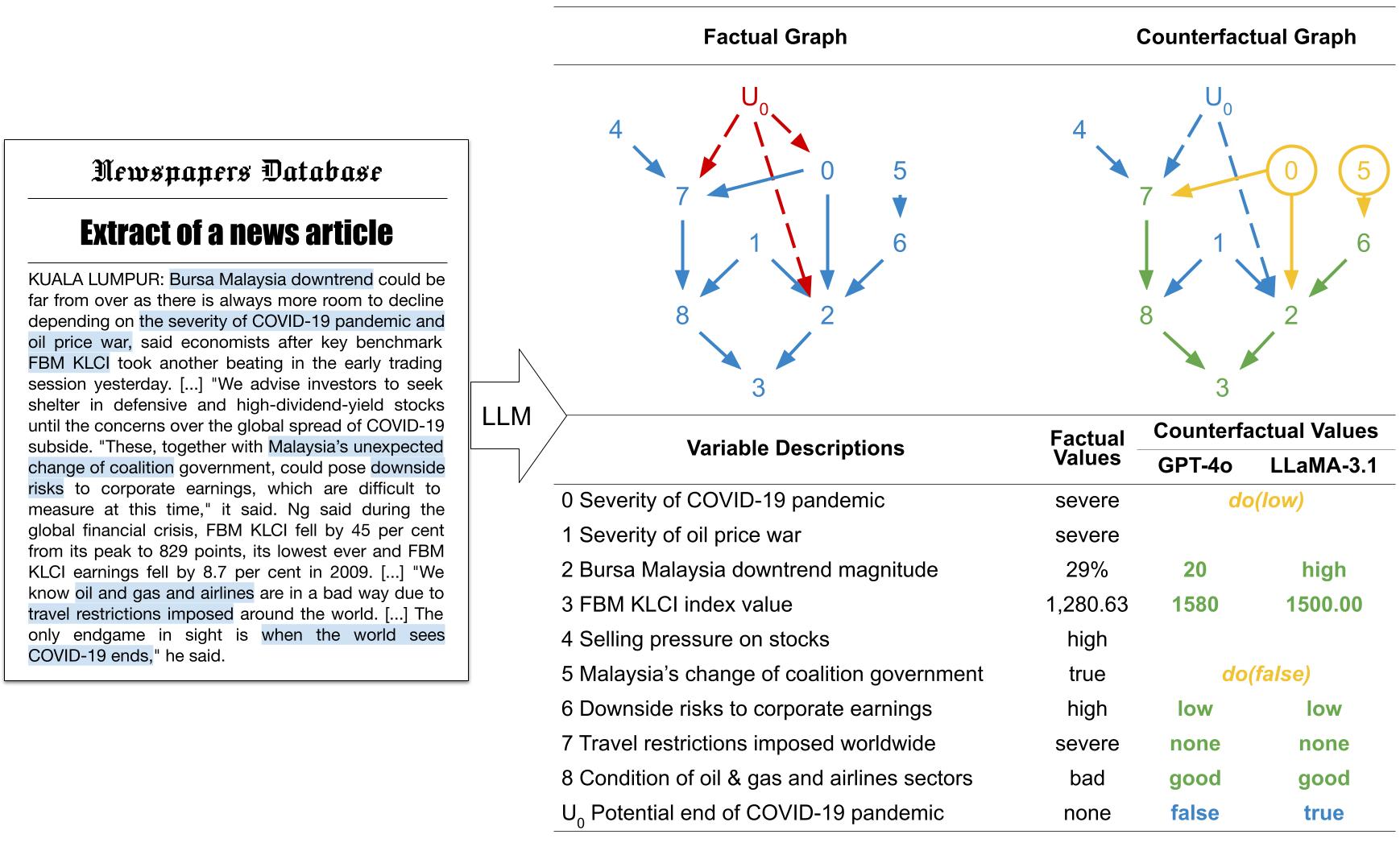
Counterfactual Causal Inference in Natural Language
We build the first causal extraction and counterfactual causal inference system for natural language, and propose a new direction for model oversight and strategic foresight.
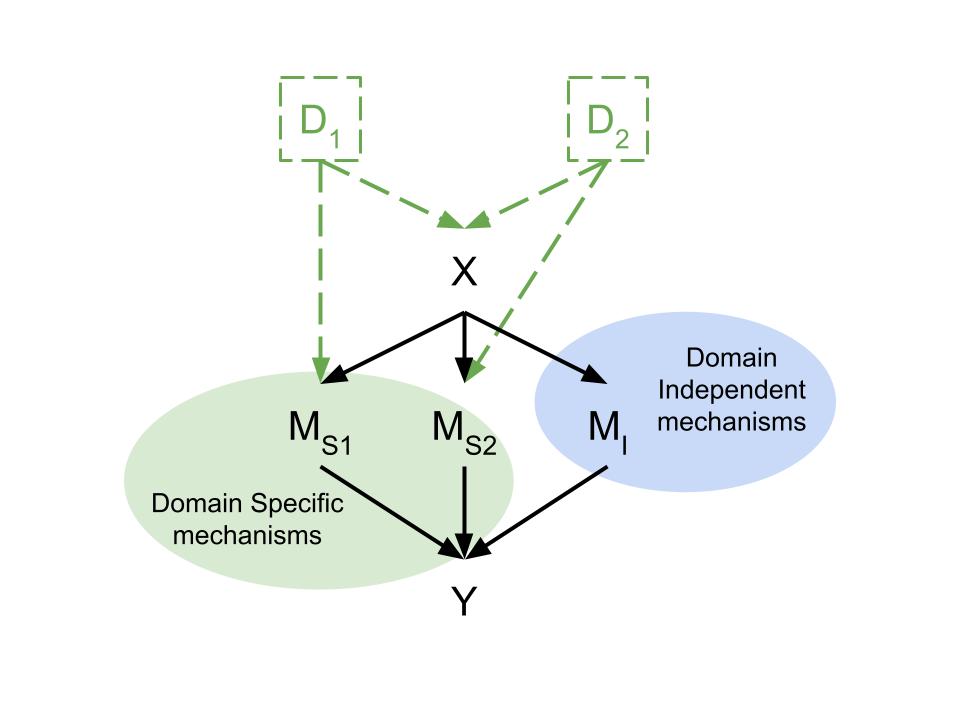
Independent Causal Language Models
We develop a novel modular language model architecture sparating inference into independant causal modules, and show that it can be used to improve abstract reasoning performance and robustness for out-of-distribution settings.
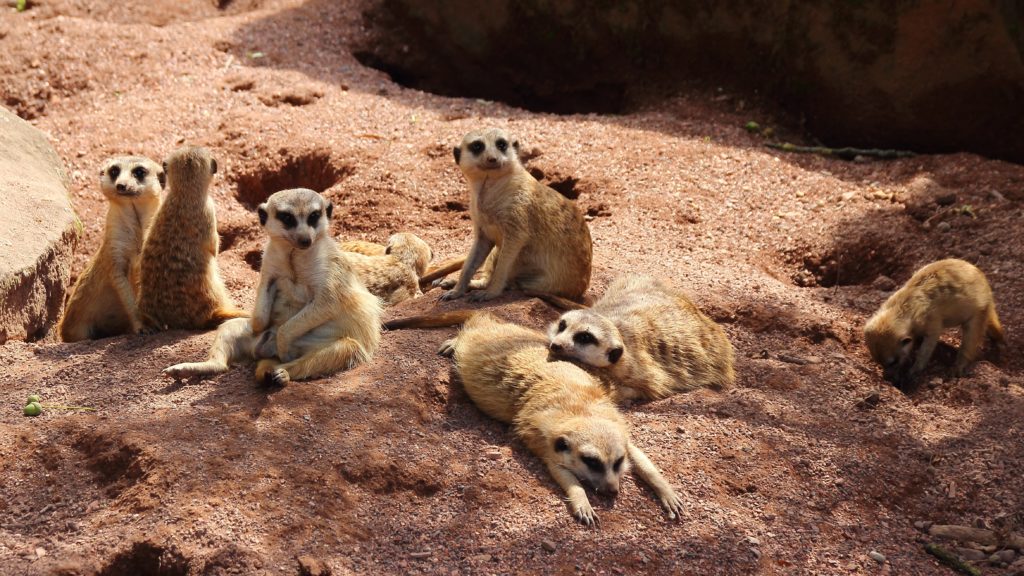
Behaviour Modelling of Social Agents
We model the behaviour of interacting social agents (e.g. meerkats) using a combination of causal inference and graph neural networks, and demonstrate increased efficiency and interpretability compared to existing architectures.

Evaluation of LLMs on Abstract Reasoning
We evaluate the performance of large language models on abstract reasoning tasks and show that they fail to adapt to unseen reasoning chains, highlighting a lack of generalization and robustness.
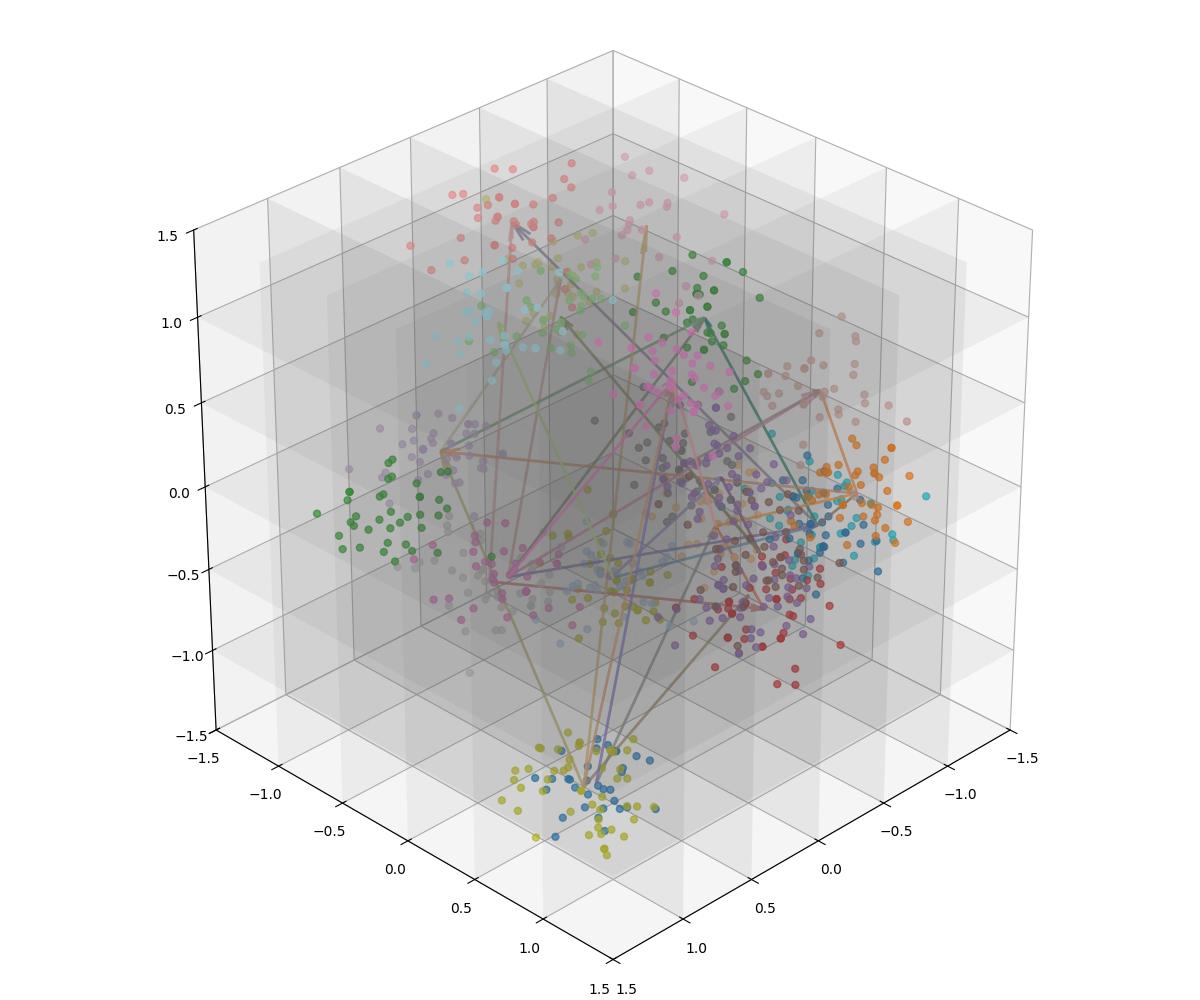
Disentanglement via Causal Interventions on a Quantized Latent Space
We propose a new approach to disentanglement based on hard causal interventions over a quantized latent space, and demonstrate its potential for improving the interpretability and robustness of generative models.